When we think of developments in artificial intelligence (AI), vision processing, autonomous vehicles, search engines and cybersecurity tend to be the first applications that come to mind. Then there are those researchers that are applying AI to meet specific industry needs, such as Atomwise. Born out of a University of Toronto research project, this startup is putting an entirely new spin on the art – and the science – of drug discovery.
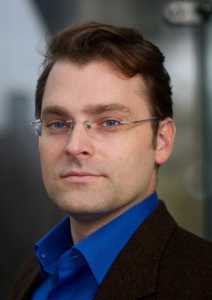
Atomwise CEO and co-founder Abraham Heifets is a computer scientist who has built a reputation on applying artificial intelligence techniques to molecular prediction. His career to date includes extensive experience in high-performance data processing at the IBM T.J. Watson Research Center. His co-founder, Izzy Wallach, worked as an algorithm developer with a drug discovery company.
“We had this idea to link our skills through AI,” Heifets explained. “We believed we could create a context between the drug discovery process and every other form of manufacturing we have on the planet.”
The idea of using computers for chemistry has been around for 40 years, Heifets said. “We’re not the first to have that idea. But applying structural 3D neural networks to biochemical data is. We’re the only company taking this particular approach right now.”
Today, in every form of manufacturing, computers play a significant role in the design, he explained. “Simulation is a very sensible approach in areas like aerospace, mechanical engineering, and cellphone manufacture where every transistor is simulated before billions of dollars are spent on production. An airplane manufacturer doesn’t design 1,000 different wings physically and test and crash them to figure out which is the best to fly. The first thing they do is simulate it.”
Atomwise’s goal is to create the same efficiencies a manufacturer or developer gains through simulation, but apply it to drug design. “Simulation informs what you build rather than the other way around,” he said. “Unlike a processor that’s designed to be understandable to people, biology is not. But that doesn’t mean you can’t use similar, predictive tools to help move drug discovery into drug design.”
In simple terms, Atomwise uses deep neural networks to do classification and prediction that can determine what molecules will “stick” and which won’t; and that perform this much faster and with greater accuracy than traditional methods. It’s easier to comprehend if you think of the body as a machine on an assembly line, he said. “When a machine breaks, production can go haywire. What you want to do is shut down that one machine, not all 100 on the factory floor. Now imagine we are talking about cell growth and division. Medicine design plugs into a region in a protein to shut that process down.”

Designing a successful medicine relies on two key properties: the drug must fit the particular protein well in order to maximize potency and minimize the dosage (to ensure safety); and it has to be selective (to improve efficacy). “You want to bind to the disease protein strongly while proteins in other parts need to keep functioning.”
The Atomwise business case speaks for itself. The dollars spent in designing a new drug are staggering; a single new medicine costs $2 billion to produce and takes 15 years, Heifets said. In one early research project, the team did a head-to-head comparison with test tube experiments by applying AI to test a disease protein. “It took them five months to do the experiment. We were able to make predictions in the same day and we ended up getting the same answer. When you are looking at a blockbuster drug, one day of sales adds up to $3 million in terms of economic consequence.”
Besides requirments in the high profile disease research areas, there is a huge unmet need in drug discovery in areas that will only get worse over time: the growing list of ‘diseases of neglect’ includes known infections such as malaria and Ebola, or newly emerging ones such as Zika or drug-resistant TB.
AI offers a fast and effective way to get the right medicines to people that need it quickly. Atomwise worked on an Ebola project in 2014, for example, that applied AI to a complete database of all known drugs and their side effects to look for a drug that could be repurposed. The idea behind drug repurposing is that all drugs have side effects – some of which can be beneficial. The classic case is Aspirin, which is now proven to have a positive impact on heart disease.
“In the Ebola case, we were looking for a medicine that had an as yet unknown side effect that could cure it. With Aspirin it was years before they discovered a beneficial side effect. With AI, it took a matter of minutes to test and come up with a short list of 17 possibilities that were shelf stable, manufacture-able, orally available and safe, and to rank them from the most to the least likely,” Heifets said. Among other projects, Atomwise is currently working with Merck on a malaria drug discovery initiative.
Because machine learning in drug discovery is infinitely more efficient, it’s now possible to develop medicines today that no one can afford to research based on other priorities, Heifets added. “It’s not just about making drug discovery cheaper or faster for what we do today; it’s about making it possible to research diseases that don’t get onto the docket at all.”