Every year there’s a tech trend that starts to dominate conversations. With announcement of NextAI, there’s a lot of buzz in Canada right now around the maturing of the machine learning ecosystem.
NextAI is a NEXT Canada initiative that is designed to bring together Canadian and international talent in the field of artificial intelligence (AI) and machine learning. The program provides qualified startups with access to technology platforms and organizational experts from Google, Microsoft, and IBM, and others who will join program. Teams will receive up to $200,000 in seed financing to develop their technology, and will also receive hands-on instruction from faculty at the University of Toronto, Georgetown University, University of Guelph, Massachusetts Institute of Technology, New York University, and Harvard University. Startups will also have access to machine learning platforms such as Google’s TensorFlow and IBM’s Watson.
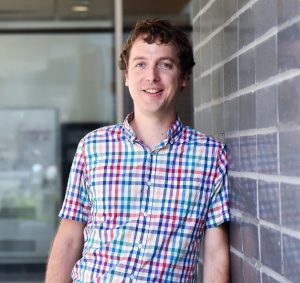
According to academic director at NextAI Graham Taylor, who also serves as assistant professor at the University of Guelph’s School of Engineering and lead for its machine learning group, “In the last few years we have seen huge gains on the side of big tech giants such as Facebook, IBM, Microsoft and Google. They have heavily invested in machine learning and built up their teams.”
So far, the work that has been done in this area has been focused mainly on Internet services and consumer electronics, where advanced functions such as Internet search, advertising and recommender systems are well down the path of applying machine learning. On the mobile side, machine learning is being used to advance speech recognition, to find the best routes on maps, for photo recognition and other tasks.
Today, machine learning is moving towards a more diverse commercialization path, Taylor said. “We’re seeing it actually spread into other verticals where there is much more diversity in applications. Now the intelligence is moving to physical environments such as robotics and vision systems. Companies like Kindred Robot [applying machine learning to bipedal walking] and Thalmic Labs [developers of gesture control armbands] are working in this space.”
One area that is set to accelerate in the not-too-distant future is self-driving cars. “This is the next big thing to hit machine learning – not just at a personal level but in shared transportation as well,” Taylor said. “Uber, for example, has bought Otto, a self-driving delivery truck system, for $680 million.”
Other applications, such as personalized medicine, may be further down the road, largely because of issues around the availability of data and the need for domain expertise. The use of drones in agriculture is yet another promising area of development.
Taylor said that while up to 500 startups in Canada are now working with machine learning, a major challenge within that community is a lack of resources to devote to the building of specialist teams. As a result, startups need to access expertise from their larger counterparts.
“There is a real shortage of talent that understands machine learning and AI,” he said. “It takes a long time for someone to get deep into machine learning and be at the top of their field. There is obviously a need to train more people.”
Since most startups can’t build their own machine learning team, larger players need to share resources, including offering up programming interface toolkits such as TensorFlow, Torch, Caffé and Theano. While “you have to be a developer to work with these,” Taylor explained, “Watson and Amazon’s proprietary platform are more general purpose, and provide user-friendly APIs that both data scientists and developers can plug into and utilize.”
What is also badly needed at this juncture is education around the terminology and use of machine learning systems, a role that NextAI is hoping to fulfill through use of a number of channels that will educate member startups at no charge.
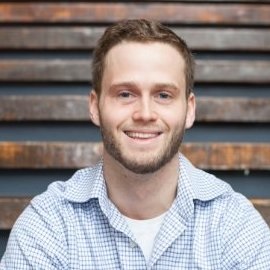
With the belief that machine learning is well on its way to being ubiquitous, Google has taken steps internally to bring staff members up to speed, said Kory Jeffrey, head of developer relations for Google Canada. “Every engineer has been given the opportunity internally to be trained on machine learning and to play with data modeling and the toolset. We are making sure our people know how to do this.”
Jeffrey also explained that the company has had its sights on expanding machine learning resources for the startup community for some time. Efforts include the establishment of developer community groups, education programs for third party engineers and access to its TensorFlow open source machine learning library.
Originally developed by researchers and engineers working on the Google Brain Team within Google’s Machine Intelligence research organization, TensorFlow was first focused on machine learning and deep neural networks research. But today, but the system is general purpose enough to be applicable in a wide variety of other domains.
“TensorFlow takes multi-dimensional arrays and allows numbers to flow through the equation rather than using brute force to get the answer,” Jeffrey explained. “It’s interesting because it’s based on neural net technology, which learns through example and gets smarter over time, as opposed to requiring pre-programming by logical rules.”
Google is sharing both the code and its research in the machine learning space to help build the startup community, Jeffrey added. “We are working with Canadian neural network pioneers such as Geoffrey Hinton and Yoshua Bengio, and spinning that research out into applications. There are some really good projects that have become really successful, such as Maluba, which involves work on natural language processing.”
Jeffrey likens the evolving machine learning ecosystem to what happened in the mobile app space in the not-so-distant past. “No one knew how to make a mobile app at first. But those who made the platforms and tools had to make sure people were given the right materials to bring these things to market.”