Microsoft is one of several major IT players racing to build the AI (Artificial Intelligence) startup community. In December 2016, the company announced a major step forward in its quest to “democratize” AI with the establishment of a new Microsoft Ventures fund specifically dedicated to funding investment in AI companies.
Microsoft Ventures’ first investment was significant for Canada: Element AI is an artificial intelligence incubator and platform located in the heart one of Canada’s technology development hubs in Montreal. According to the investment announcement, Element AI funding was designed to support Element AI’s role in helping large corporations, world-class entrepreneurs and researchers solve hard problems with AI.
It also stated that Microsoft’s move into AI expands its original mandate to support cloud companies that help enterprises navigate digital transformation, and noted the expectation that the pace of investments will accelerate.
That acceleration quickly became apparent this January with Microsoft’s acquisition of Maluuba, a Montreal-based deep learning research lab whose focus is on natural language understanding. Maluuba will now be an integral part of Microsoft’s global network of AI research and development organizations. In addition, Microsoft has announced gifts for AI research: $6 million to the Université de Montréal and $1 million to McGill University to be funded over a five-year period.
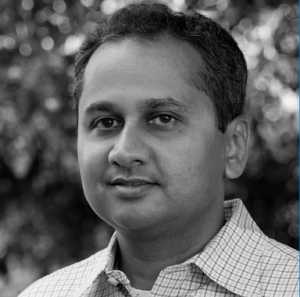
“All this activity is a result of our being in a position where industry has the data and processing power, but too little talent,” said Nagraj Kashyap, corporate VP of Microsoft Ventures. “Through Element AI, we can get data access and solve key technology problems for businesses that they can’t solve on their own.”
Kashyap compared AI activity in Canada to Israel’s extensive AI research efforts in cybersecurity. “Now there’s a big R&D hub there. In Canada, the initial focus has been on the part of telecom companies. Early demand is coming from them, because they have lots of data they can apply deep learning to in order to automate many aspects of their operations, including call centres.”
The push to support the R&D and incubator community is not aimed at replacing human talent, but rather augmenting it, he stressed. “Take cybersecurity as an example. The industry is looking at net negative unemployment: anywhere from 200,000 to 400,000 positions in North America can’t be filled. AI can effectively augment humans rather than replace them and make operations more efficient. Then there are areas like genomics and medicine where clearly the amount of data is huge. There is simply no way humans can go through it on their own.”
Although AI research actually dates back to the 1980s, the current explosion of activity in this space is a result of the convergence of two aspects that are critical to deep learning: the availability of data and massive compute cycles, he explained. “The need for both is the reason why this has only taken hold in the last five years. First the availability of data in corporations combined with what we generate every day through phones, videos and photos demands a massive computing infrastructure.”
That grand scale has been realized through the emergence of the public cloud. “That is what has made it possible to harness an amazing amount of compute power,” Kashyap said. “The public cloud allows organizations to solve deep learning problems without having to have a compute capacity infrastructure.” In fact, Microsoft CEO Satya Nadella stated last fall in Dublin that Azure is on its way to becoming the first artificial intelligence supercomputer.
Azure’s capacity exceeds one exaflop and can process one billion billion operations a second. That is equivalent to the ability to translate the entire English Wikipedia opus – five million articles and three billion words – into another language in less than one-tenth of a second. “That’s the kind of computing power we can throw at a problem today,” Kashyap said. “We just have to unlock the data and solve the talent issue.”
While unable to share details, Kashyap said that Microsoft research has a variety of platforms under development that they will expose so other researchers can use them. “We have always focused on democratizing AI. The way it is today it is simply not sustainable. If platforms can be used by more people beyond the PhDs, and encourage collaborative research with other companies, we can collectively advance AI so more developers can use it. We all want to make sure that the benefits of AI are not limited to a few people.”
Mobile app development is a case in point. Platforms were fragmented, and early development efforts took time, effort and a great deal of customization, he explained. “Now anyone can build a mobile app and put it in an app store. Of course we are clearly not anywhere close to that on the AI front; but we will consider it a win if any developer can work with it without having to have a PhD. That will be very powerful.”
The first order of the day is to build the next set of developers and IT that can tap into the power of AI. “Only deep learning researchers and developers can do that today. We need to hide the complexity that goes into building deep learning models and continue to build access for ordinary developers. If they can start tapping into the power of AI it will be a big win for industry. It’s clearly a matter of when, not if.”